Introduction
In our previous article, we covered how to build a ChatGPT-like platform using BERT, Python, and React. In this article, we will create a similar platform using GPT-2, a powerful generative language model developed by OpenAI. We'll assume you've already read the previous article and are familiar with the concepts discussed therein.
Preparing the GPT-2 Model
Loading a pre-trained GPT-2 model
To load a pre-trained GPT-2 model, we'll use the Hugging Face Transformers library. Here's a code snippet for loading a pre-trained GPT-2 model:
This code snippet imports the necessary classes from the Hugging Face Transformers library, specifies the GPT-2 model name, and loads both the tokenizer and the model itself. The tokenizer is responsible for converting input text into a format the model can understand, while the model generates the output based on the given input.
Fine-tuning the GPT-2 model for a chatbot
To fine-tune GPT-2 for a chatbot, you'll need a dataset with conversational data. For this tutorial, we will assume you have a dataset in the form of a list of input-output pairs. Here's a code snippet to fine-tune GPT-2 using this dataset:
This code snippet sets up the GPT-2 model, tokenizer, and configuration using the Hugging Face Transformers library. Then, it creates a custom dataset for training using the TextDataset
class. The dataset should be a text file where each line represents a conversation turn (alternating between user input and chatbot response).
The DataCollatorForLanguageModeling
class is used to prepare the training data for the language modeling task, and TrainingArguments
class is used to configure the training settings, such as output directory, number of epochs, batch size, and checkpoint saving frequency.
Finally, the Trainer
class is instantiated with the model, training arguments, data collator, and training dataset. The train()
method of the Trainer
class is then called to fine-tune the GPT-2 model on the chatbot-specific dataset.
Once fine-tuning is complete, you can save and load the fine-tuned model using the following code snippet:
After fine-tuning, you can use the model to generate chatbot-like responses as described in the previously explained code snippets for setting up a Flask API and building a React frontend.
Building the Backend with Python
Creating an API endpoint for the chatbot
We'll reuse the Flask API from the previous article. First, make sure Flask is installed:
Next, set up the API endpoint:
Integrating the GPT-2 model with the API
Now let's integrate the fine-tuned GPT-2 model with the API. Replace the chat()
function with the following code snippet:
Scraping and tokenizing
We'll reuse the scraping and tokenizing code snippets from the previous article. Here's a code snippet for scraping and tokenizing text using BeautifulSoup, requests, and nltk:
Building the Frontend with React
Creating a simple chatbot UI
We'll reuse the React UI from the previous article. To set up a new React app, run the following command:
Next, open src/App.js
and replace its content with the following code snippet to set up a simple chatbot UI:
Connecting the front-end to the backend
To connect the front-end to the backend, use the Fetch API to send user input to the backend and display the chatbot's response. Replace the handleSend
function with the following code snippet:
Conclusion
In this article, we've guided you through building a ChatGPT-like platform using GPT-2, Python, and React. We've covered loading and fine-tuning a pre-trained GPT-2 model, creating a Flask API, integrating GPT-2 with the API, building a simple React frontend, and deploying the platform. We encourage you to experiment with different models and fine-tuning approaches to improve your chatbot's performance. Remember, continuous learning and development are essential in the fast-paced AI field. Good luck with your ChatGPT-like platform!
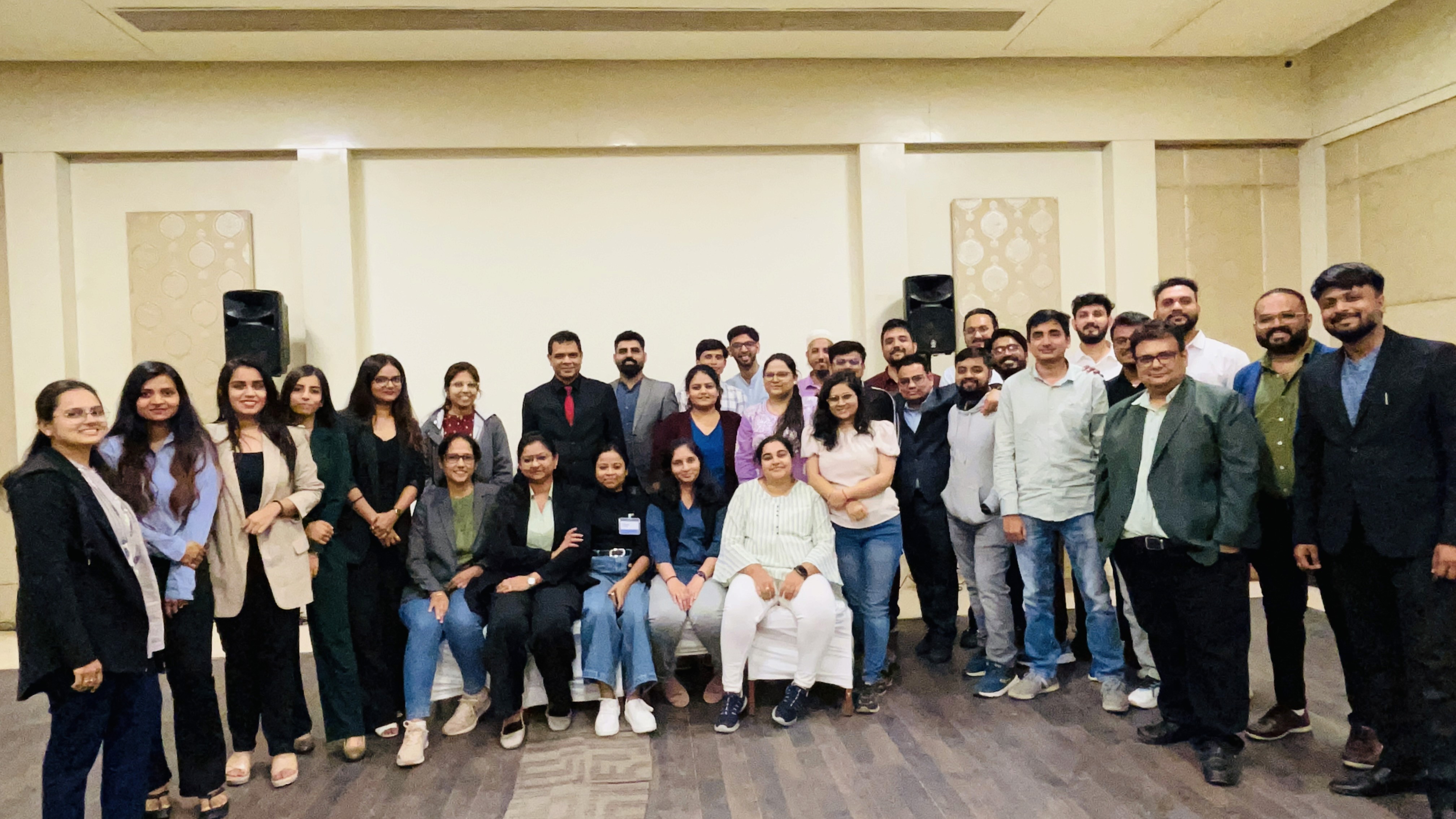
We are a family of Promactians
We are an excellence-driven company passionate about technology where people love what they do.
Get opportunities to co-create, connect and celebrate!
Vadodara
Headquarter
B-301, Monalisa Business Center, Manjalpur, Vadodara, Gujarat, India - 390011
Ahmedabad
West Gate, B-1802, Besides YMCA Club Road, SG Highway, Ahmedabad, Gujarat, India - 380015
Pune
46 Downtown, 805+806, Pashan-Sus Link Road, Near Audi Showroom, Baner, Pune, Maharashtra, India - 411045.
USA
4056, 1207 Delaware Ave, Wilmington, DE, United States America, US, 19806
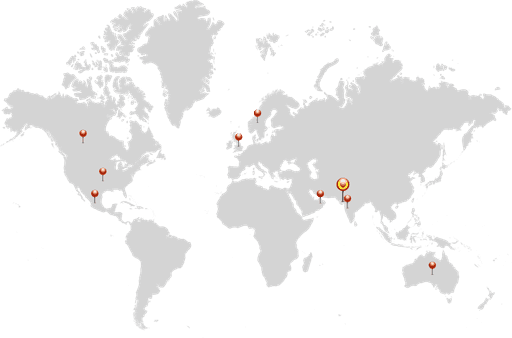
Copyright ⓒ Promact Infotech Pvt. Ltd. All Rights Reserved
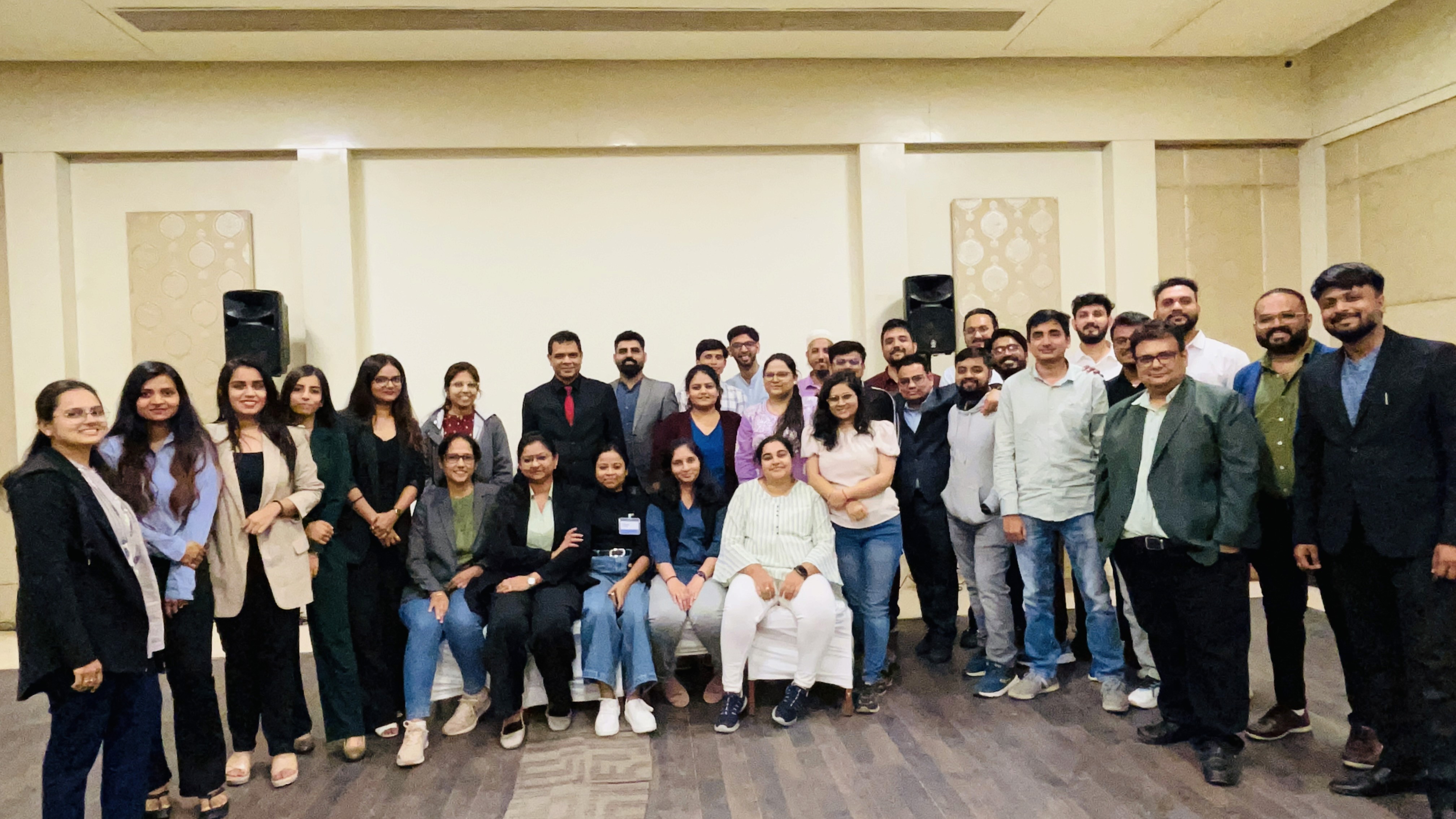
We are a family of Promactians
We are an excellence-driven company passionate about technology where people love what they do.
Get opportunities to co-create, connect and celebrate!
Vadodara
Headquarter
B-301, Monalisa Business Center, Manjalpur, Vadodara, Gujarat, India - 390011
Ahmedabad
West Gate, B-1802, Besides YMCA Club Road, SG Highway, Ahmedabad, Gujarat, India - 380015
Pune
46 Downtown, 805+806, Pashan-Sus Link Road, Near Audi Showroom, Baner, Pune, Maharashtra, India - 411045.
USA
4056, 1207 Delaware Ave, Wilmington, DE, United States America, US, 19806
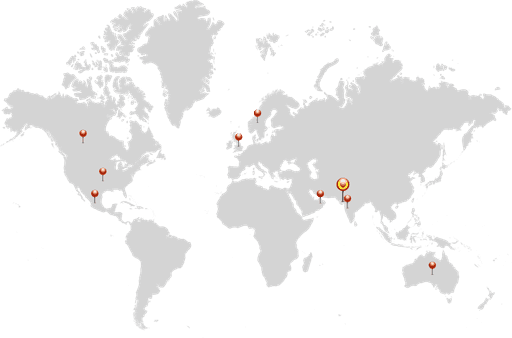
Copyright ⓒ Promact Infotech Pvt. Ltd. All Rights Reserved